What is Model Autophagy Disorder?
Are you curious as to what Model Autophagy Disorder (MAD) is and why it's a growing concern? We got you covered.
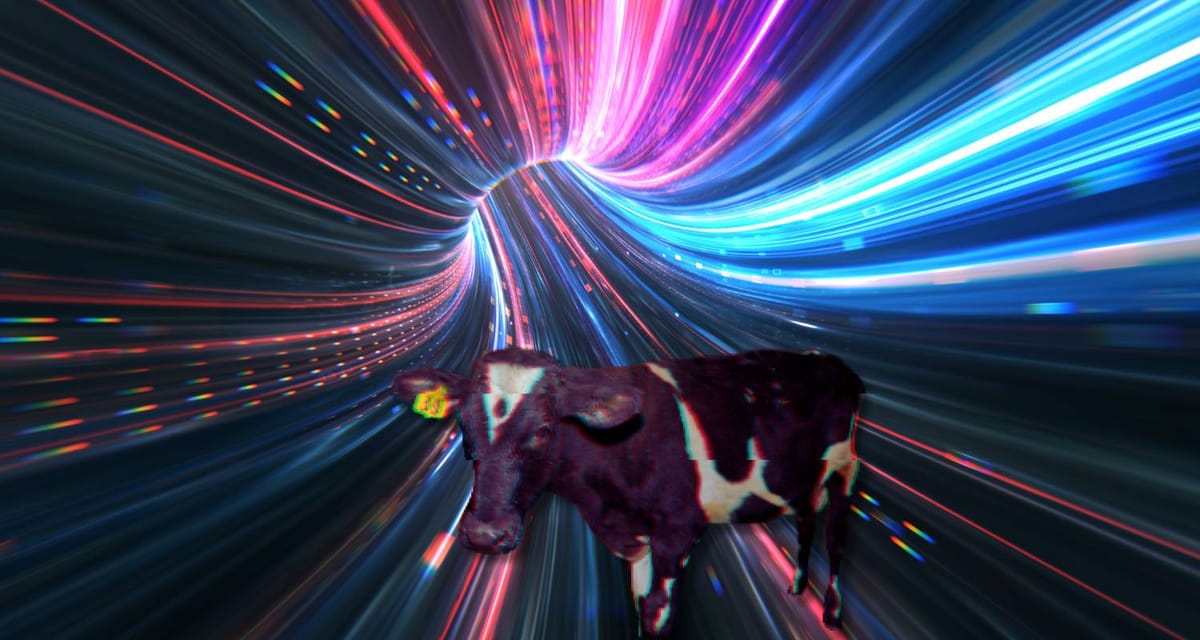
Model Autophagy Disorder (MAD) is a growing concern in the world of AI development. It describes a phenomenon where machine learning models degrade over time due to "data inbreeding"—a scenario where AI systems are trained on datasets made up largely of outputs from other AI models instead of diverse, real-world data.
Why Is It Called "Model Autophagy Disorder"?
The term draws a parallel to mad cow disease, where cows developed a fatal condition after consuming processed byproducts from their own species. Similarly, MAD occurs when AI models "consume" their own outputs or those of similar systems, leading to degraded performance over time and a loss of fidelity to real-world complexities.
Key Characteristics of MAD
- Recursive Training: Models are repeatedly trained on synthetic AI-generated data, distancing themselves from real-world contexts.
- Loss of Diversity: Training data becomes homogenized, lacking the variability necessary for accurate, generalizable outputs.
- Quality Degradation: Models struggle to handle complex or nuanced tasks as they "forget" the richness of real-world data.
Why Does MAD Matter?
- Performance Issues: Models affected by MAD produce less accurate results, struggling with tasks that require nuanced understanding.
- Reliability Risks: Flawed AI systems can lead to trust issues, particularly in critical industries like healthcare or legal tech.
- Feedback Loops: As AI-generated content grows online, the risk of recursive training intensifies, creating a self-perpetuating cycle of degradation.
Potential Solutions
- Diversify Data Sources: Prioritize real-world, high-quality datasets over synthetic ones to ensure training data remains robust.
- Regular Model Testing: Continuously monitor models for signs of degradation and take corrective action when necessary.
- Synthetic Data Quality Control: When using AI-generated data, ensure it’s diverse, accurately labeled, and representative of real-world conditions.
How MAD Relates to Habsburg AI
MAD and the concept of Habsburg AI address a similar issue—recursive training on AI-generated data—but use different metaphors to illustrate the risks.
- Habsburg AI: Draws inspiration from the inbreeding of the Habsburg dynasty, highlighting how lack of diversity weakens a system over generations.
- MAD: Focuses on the immediate consequences of recursive training, likening the degradation to a systemic disorder like mad cow disease.
Both concepts stress the dangers of over-relying on synthetic data, leading to homogenized, less reliable AI models. Together, they underline the importance of diverse and real-world datasets to maintain AI's robustness and relevance in an ever-evolving world.
The Takeaway
Knowing what Model Autophagy Disorder is (and why it matters) is a big deal as AI shapes our future. The fix? Keep your data diverse, check up on your models like they’re overdue for a doctor’s visit, and don’t let AI feast on its own leftovers.
Do that, and we’ll keep AI sharp, reliable, and ready for whatever comes next.