Jevons Paradox: The 150-Year-Old Idea Explaining AI’s Energy Problem
Jevons Paradox. What is it, and why is everyone in AI talking about it?
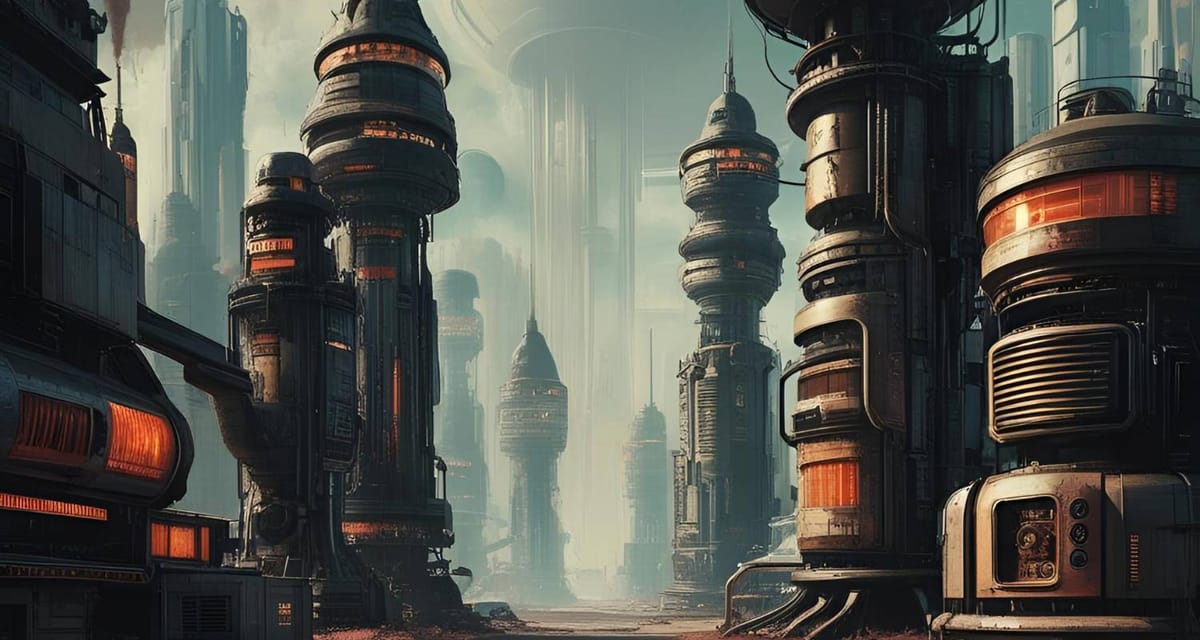
As artificial intelligence becomes more efficient, intuitive, and accessible, many believe we’re heading toward a world where machines do more with less. But there’s an old economic principle that flips this narrative on its head. It’s known as Jevons Paradox.
So what is Jevons Paradox, and why does it matter for AI? Let’s break it down.
What is Jevons Paradox?
Jevons Paradox is an economic theory introduced by British economist William Stanley Jevons in 1865. He observed that as coal-powered steam engines became more efficient, Britain didn’t use less coal — it used more. Why? Because efficiency lowered costs and increased demand.
In short:
Increased efficiency doesn’t necessarily reduce resource consumption — it can actually increase it.
This counterintuitive idea applies to a surprising number of industries, from transportation to agriculture - and now, to artificial intelligence.
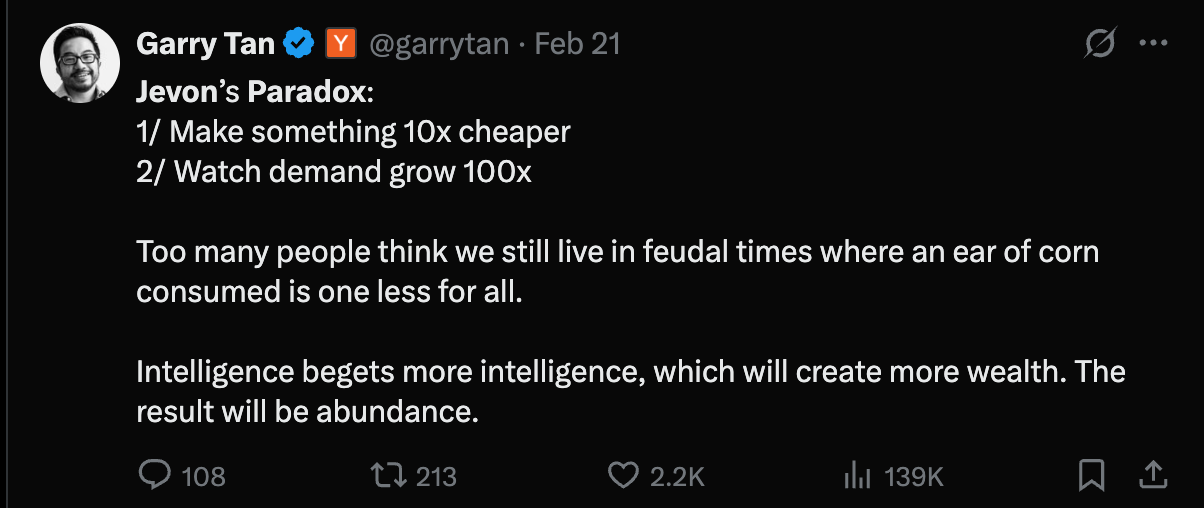
Applying Jevons Paradox to AI
AI systems are getting faster, cheaper, and more energy-efficient. Tools like GPT models, AI copilots, and generative algorithms are becoming integrated into everything from coding workflows to customer support to biotech research.
But instead of curbing resource use, the opposite might be happening:
- More models are being deployed because they’re easier to run.
- AI-powered apps are multiplying because it’s now affordable to bake AI into everything.
- Compute usage is rising fast, even as models get more efficient.
That’s Jevons Paradox in action.
Examples of Jevons Paradox in AI
1. Cheaper inference, more usage
As inference costs drop (thanks to hardware acceleration and model optimization), developers start embedding AI into every product. An example is when ChatGPT launched image generation. The result? Skyrocketing demand for compute.
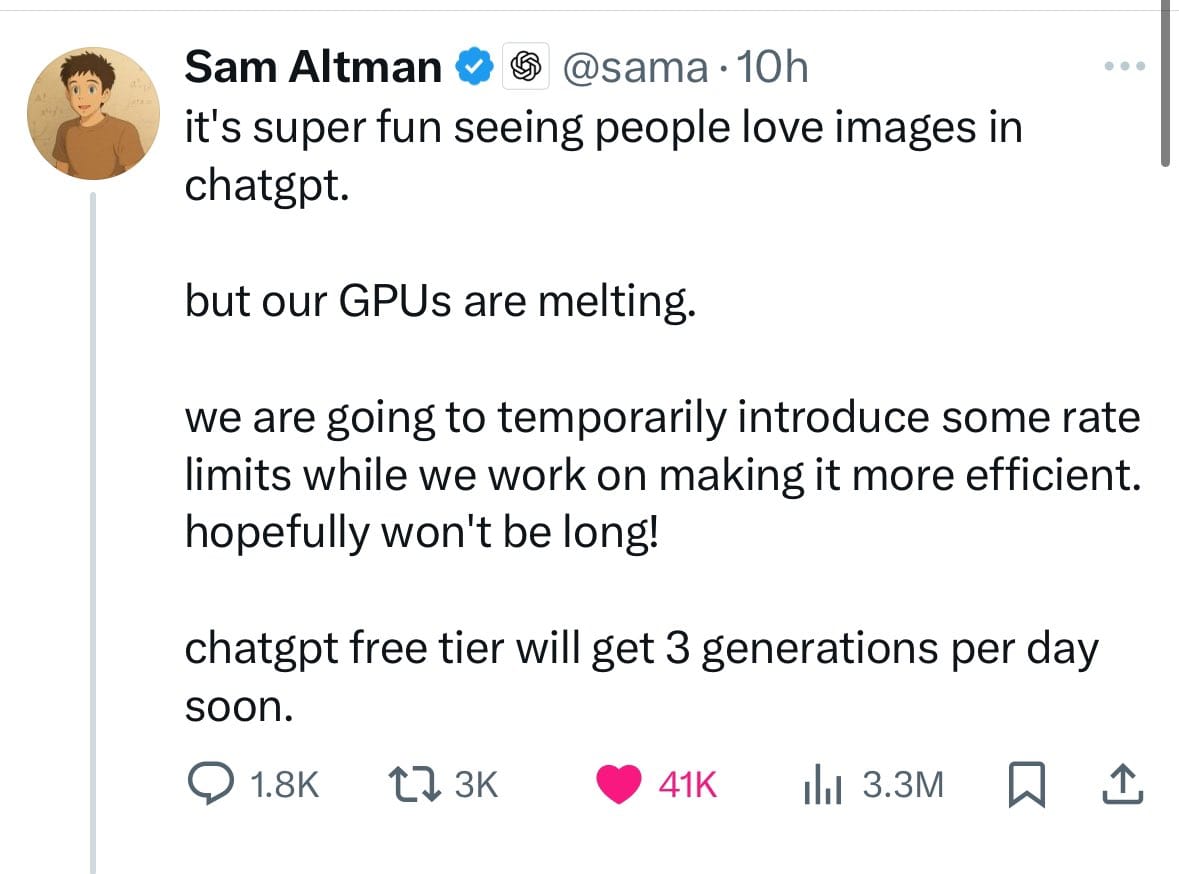
2. Edge AI and IoT
Edge AI promises to reduce cloud reliance by running models locally on devices. But as it becomes easier to embed AI in smart cameras, sensors, or wearables, we’re deploying more devices.
3. Open-source models and accessibility
The rise of open-source AI models like LLaMA or Mistral has lowered the barrier for startups and indie developers. This has sparked a wave of new products and services, increasing the overall consumption of compute resources, bandwidth, and storage.
What Jevons Paradox Means for AI Sustainability
At first glance, making AI more efficient seems like the right move for sustainability. However, if efficiency leads to more total usage, energy consumption could continue rising.
This means we can’t rely on model efficiency alone to reduce AI’s environmental impact. Instead, we need:
- Smarter usage incentives (e.g., green AI credits or carbon-aware scheduling)
- Hardware innovation to reduce per-inference energy use
- Governance policies that guide sustainable AI deployment at scale
Final Thoughts
Jevons Paradox reminds us that efficiency is not the same as reduction.
As AI becomes cheaper, faster, and more widely available, total demand for computing, energy, and infrastructure will likely keep rising.