AI Observability: The Key to Keeping AI Reliable and Cost-Effective
Let’s dive into AI Observability. What it is, why companies are prioritizing it, and what companies are leading the charge in this space.
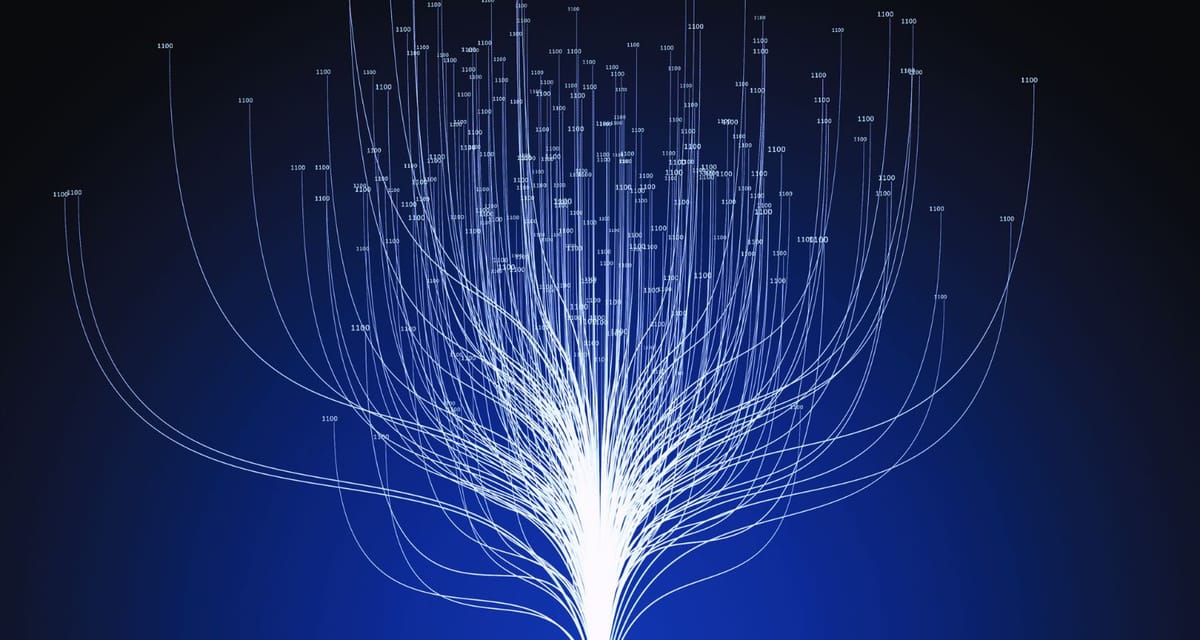
For many businesses, AI remains a mysterious black box. How can you ensure your AI models perform at their best, do not drain your budget, and do not silently fail when you're not looking?
This is where AI observability comes into play. Think of it as the eyes and ears performance to ensure reliability and cost-efficiency.
What Is AI Observability?
AI observability is the continuous monitoring and analysis of AI models and data pipelines. Think of it as a comprehensive health check for your AI systems that:
- Detects and fixes performance issues before they become major problems
- Prevents hidden costs from quietly draining your enterprise budget
- Ensures your AI systems remain trustworthy, explainable, and compliant
Spotting failures is one piece. The other is proactively preventing them.
Why Your Enterprise Needs AI Observability
AI models aren't static. They evolve, adapt, and sometimes break in ways you might not expect. Without proper observability, your organization could face:
- Skyrocketing cloud costs – Running AI models can cost millions if you're not tracking expenses
- Trust challenges – Did you know 80% of AI proof-of-concepts stall because users don't trust the results?
- Hidden inefficiencies – Your data pipelines might be full of inefficiencies that waste valuable resources
- Trust challenges – A strong AI governance framework can help solve this—learn more about AI governance here.
Key Metrics Worth Tracking
AI observability keeps an eye on:
- Model Performance – Is your AI accurate, reliable, and efficient?
- Data Pipeline Efficiency – Are you optimizing storage, queries, and processing?
- Latency & Uptime – Is your AI system fast and available when you need it?
- Cloud & Compute Costs – Where might you be overspending on AI infrastructure?
The good news is that there are companies looking to solve this problem!
Companies Leading the Charge
Several innovative companies are developing cutting-edge AI observability tools:
- Unravel Data – Specializes in AI observability and data FinOps to optimize AI model costs
- WhyLabs – Focuses on AI model monitoring to detect performance degradation and bias
- Arize AI – Provides observability for ML models, detecting drift and optimizing performance
- Weights & Biases – Offers ML experiment tracking and performance monitoring for AI teams
The Future Looks Bright
As enterprises continue investing heavily in AI, observability will become a core requirement, not just a nice-to-have option. Without it, companies risk uncontrolled costs, unreliable models, and AI failures that could damage trust and revenue.
Remember: AI is only as good as its monitoring and maintenance and AI observability is what keeps everything running smoothly!