Retrieval Augmented Generation: How AI Is Getting Smarter
Are you a non-technical person looking to understand Retrieval Augmented Generation (RAG)? You're in the right place!
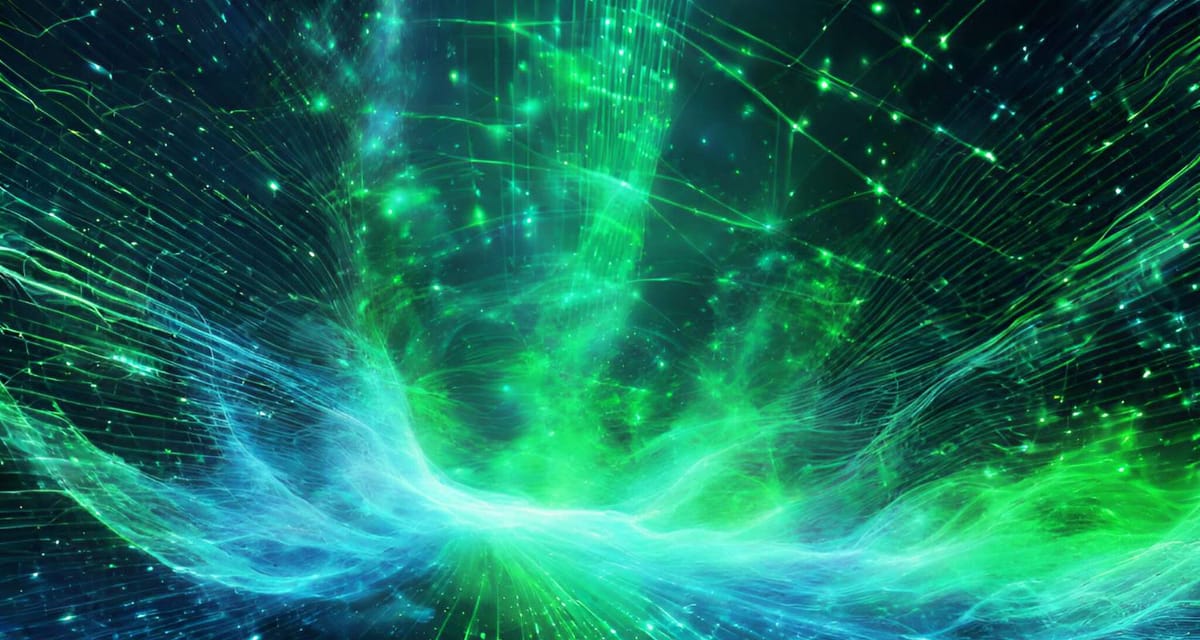
Are you a non-technical person looking to understand Retrieval Augmented Generation (RAG)?
You're in the right place!
What is Retrieval Augmented Generation? - The Basics
Retrieval Augmented Generation (RAG) is a framework for improving the results of a large language model (LLLM).
Imagine this: while traditional text generators are stuck playing with their blocks of pre-learned patterns, RAG pulls fresh data into the mix live, as it writes. It’s not just regurgitating facts; it’s making them dance to the tune of the current context.
This framework of retrieving and generating elevates the whole text game, delivering content that’s not just accurate but also a heck of a lot more riveting.
How Retrieval Augmented Generation Works
Here’s the rundown on how this magic happens:
- Query Generation from Input: It all starts with the input. First, analyze the user-provided text to understand the query's context. This initial step helps set the direction for the information retrieval process.
- Document Retrieval from a Database or Knowledge Base: Once the query is defined, RAG taps into an extensive database or a structured knowledge base to fetch relevant documents or data snippets. This retrieval is guided by the initial query, ensuring the information sourced aligns with the user's needs.
- Content Generation Using Retrieved Information: With the relevant information at hand, the RAG model then shifts gears to the generation phase. Here, it weaves the retrieved data into the final text output, ensuring the information is integrated seamlessly and naturally.
- Integration of Retrieval and Generation Components: The true magic of RAG lies in the integration of its retrieval and generation components. This integration allows the system to refine its output continuously by cross-checking with the retrieved information, ensuring accuracy, and enhancing the richness of the content.
By dynamically interacting with external data sources, RAG systems can generate more accurate, informative, and contextually relevant content than ever before, marking a significant leap forward in the capabilities of generative AI systems.
Components of RAG
Remember the components we mentioned above? Those are the retriever and the generator. Each plays a role in the process, ensuring the output is relevant and well-articulated.
Here’s a closer look at each:
- The Retriever: The retriever is essentially the fact-finder of the RAG system. Its primary task is to sift through vast amounts of data—documents, databases, etc, to find information that matches the input query. This component uses algorithms to understand the query's context and select relevant and credible data. It’s like having a highly efficient librarian who knows exactly where to find the perfect piece of information for any topic under the sun.
- The Generator: Once the retriever has fetched the necessary data, the generator performs its magic. This component takes the retrieved information and crafts it into coherent and contextually appropriate text. The generator’s ability to synthesize pieces of information into a seamless narrative is what sets RAG apart from simpler text generation models. It’s akin to a skilled storyteller who can weave facts into a narrative that not only informs but also captivates the audience.
Together, these components help RAG systems produce content that is rich in information and highly tailored to the user's specific needs.
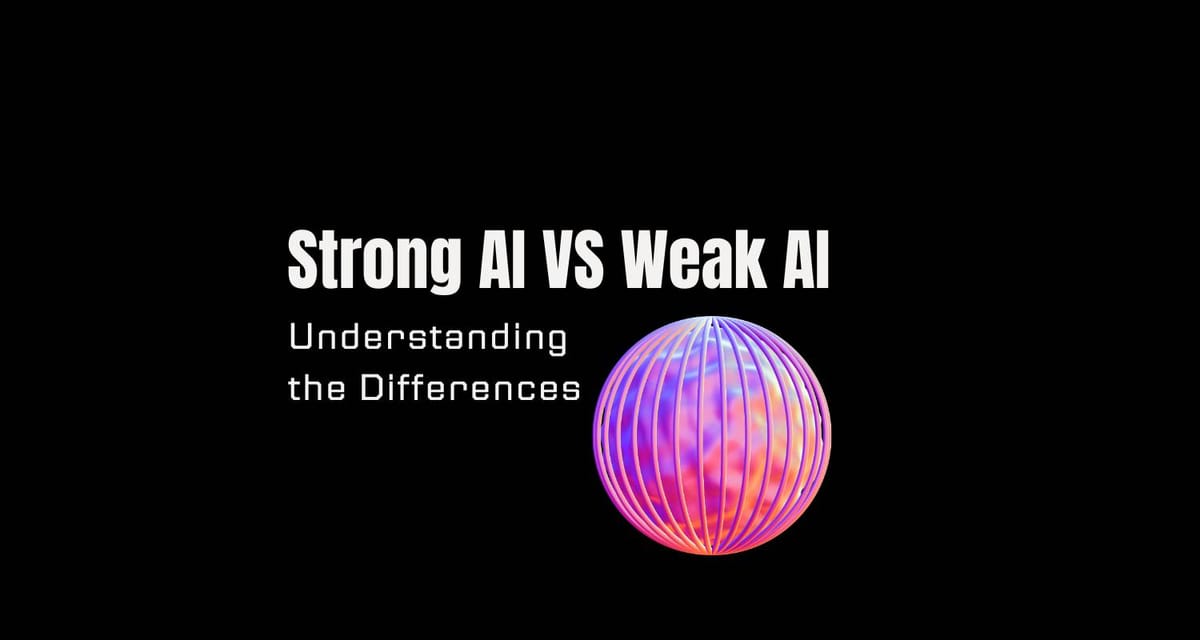
Digging Trends in RAG
As RAG keeps pushing the envelope, new trends are making waves, tweaking how it works and where it can make a splash.
Let's dive into some of the hottest trends:
- Chain of Thought Prompting: This involves crafting prompts that guide the AI through a logical sequence of thoughts before arriving at a conclusion. It's like giving the AI a breadcrumb trail of thoughts to follow before it hits you with an answer. This method helps RAG to churn out responses that aren't just accurate but are thoughtfully pieced together, much like a human solving a puzzle step by step.
- Synthetic Data Generation: Ever run into a data drought? Well, RAG's got a trick up its sleeve. This involves creating artificial datasets that closely mimic real-world data, allowing models to learn and perform in scenarios where actual data may not be available.
- Few-Shot Prompting: This is all about getting RAG to do more with less. Few-shot prompting trains systems to perform effectively by seeing just a few examples, enhancing the model's ability to generalize from limited data and reducing the need for extensive training datasets.
- Data Augmentation: Here's where RAG systems turn a little data into a lot. It takes existing datasets and expands them by spinning out variations, beefing up the model's accuracy and resilience. This not only helps in avoiding the dreaded overfitting but also gears up RAG to tackle a broader array of queries with gusto.
Each of these trends is shaping RAG into a sharper, more adaptable tool, ready to tackle tasks across various domains with a fresh flair.
Challenges and Considerations in Retrieval Augmented Generation
Implementing Retrieval Augmented Generation systems comes with its own set of technical and ethical challenges that need careful navigation.
Here's a deeper look into these challenges:
- Data Quality and Relevance: The backbone of any RAG system is the data it pulls from. If the data’s dodgy, the output will most likely be too. Ensuring the information is top-notch and relevant is critical because let's face it, garbage in, garbage out. Skewed or low-quality data can make a RAG system spit out content that's about as reliable as a chocolate teapot.
- Privacy Concerns: With RAG systems tapping into massive pools of data, the privacy red flags are waving. It’s vital these systems don't just play fast and loose with privacy norms, especially when handling sensitive info. Keeping on the right side of privacy laws and ethical lines isn’t just nice—it’s non-negotiable to keep users’ trust and dodge legal hot water..
- Computational Costs: These systems are like the gas-guzzlers of the computing world, hungry for processing power to sift through data mountains efficiently. This can crank up the costs, particularly when you need lightning-fast content generation. Striking a balance between zippy performance and not blowing the budget is a real brain teaser.
- Ethical Considerations: Then there's the moral maze—ensuring RAG doesn’t end up dishing out skewed info. Keeping a tight leash on algorithms is key to ensuring they don’t mirror any unfair biases.
As RAG systems evolve, the playbook on managing these challenges needs to be dynamic, making sure these smart systems are also wise to the world's ways.
Conclusion
Wrapping up, RAG isn’t just another step forward in AI; it’s a giant leap for machine-kind. It sets the stage for a future where AI could turn our data dumps into gold mines of useful content, making knowledge more accessible and sparking even more creativity.
Strap in, because the AI ride is only getting started!